Estimation of Surface Roughness in Selective Laser Sintering Using Computational Models
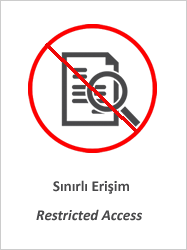
View/ Open
Access
info:eu-repo/semantics/embargoedAccessDate
2022Author
Koç, EbubekirZeybek, Sultan
Kısasöz, Burçin Özbay
Çalışkan, Cemal İrfan
Bulduk, Mustafa Enes
Metadata
Show full item recordCitation
KOÇ, Ebubekir, Sultan ZEYBEK, Burçin ÖZBAY KISASÖZ, Cemal İrfan ÇALIŞKAN & Mustafa Enes BULDUK. "Estimation of Surface Roughness in Selective Laser Sintering Using Computational Models". The International Journal of Advanced Manufacturing Technology, 123 (2022): 3033-3045.Abstract
This study presents a comprehensive experimental dataset and a novel classification model based on Deep Neural Networks
to estimate surface roughness for additive manufacturing. Many problems exist due to the very complex nature of the production
process. Some focus on the production planning phase, including the nesting problem under many constraints. However,
it is not possible to solve the main function without a clear understanding of the nature of the constraints. The purpose of
this research is to present a method to automate the surface roughness estimation process in the production planning phase.
The significance of this study is to implement a data-driven model for one of the most critical decision constraints in the
nesting process. Solving this problem will automate a key decision constraint, and it might be implemented as an automated
constraint module in solving the nesting problem. The proposed model focused on selective laser sintering (SLS) technology
based on polyamide 12 powder applications. A comprehensive dataset is designed to simulate the behaviour of an industrial
SLS manufacturing process based on a 3D positioning strategy. A set of samples with random positions are also created
to test present the model’s robustness. The proposed classification model is based on Deep Neural Networks (DNN) with
hyper-parameters designed for the problem. The dataset and the model provide a new user interface to estimate the surface
roughness depending on the coordinates of a given product surface in an SLS production chamber and the production parameters
employed in the production planning phase. The results show that the model can classify sample surfaces as “rough” or
“smooth” with a very high percentage (95.8%) for the training set and with 100% for the test set. Benchmark results also show
that the model outperforms other machine learning methods in classifying the surface roughness successfully on the test set.