Mechanical Performance and ANN-Based Prediction of Co-Cr Dental Alloys with Gyroid Cellular Structures Produced by LPBF Technology
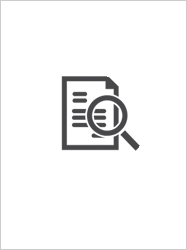
View/ Open
Access
info:eu-repo/semantics/embargoedAccessDate
2025Author
Çalışkan, Cemal İrfanŞahin, Meryem
Koca, Aliihsan
Tarakçı, Gürkan
Okbaz, Abdulkerim
Dalkılıç, Ahmet Selim
Metadata
Show full item recordCitation
ÇALIŞKAN, Cemal İrfan, Meryem ŞAHİN, Aliihsan KOCA, Gürkan TARAKÇI, Abdulkerim OKBAZ & Ahmet Selim DALKILIÇ. "Mechanical Performance and ANN-Based Prediction of Co-Cr Dental Alloys with Gyroid Cellular Structures Produced by LPBF Technology". Proceedings of The Institution of Mechanical Engineers, Part C: Journal of Mechanical Engineering Science, (2025): 1-19.Abstract
Gyroid structures exhibit significant potential in the fields of lightweight structural design, heat transfer, energy
absorption, and biological applications. The use of gyroid for implants in dentistry is currently not sufficiently
widespread. The research encompasses design, compression testing, cellular investigation using a digital microscope,
and the application of artificial neural networks (ANNs) using data gained from the compression test. The ANN study
and the test phase in which gyroid geometries are addressed by dental three-point bending tests are novel in this field.
In the field of dentistry, this study compares the usability of five distinct gyroid design characteristics, including one
model without a gyroid structure. During the testing, we found that the m1 model had an average maximum strength
of 600 N, while the m3 model achieved 230 N. The remaining models achieved an approximate strength of 200 N. In
the mechanical performance evaluation of the samples, a 40% weight reduction was achieved. An ANN model has been
developed to predict the force experienced by gyroid structures under certain deformations depending on the infill
ratio. This model was trained with data obtained from a three-point bending test. Using grid search and Monte Carlo
cross-validation, the optimal multilayer perceptron structure was determined to have 12 neurons in the hidden layer, a
mini-batch size of 8, and a learning rate of 0.0001. The Adam optimization algorithm was used to train the ANN
model, which was constructed using the TensorFlow library. Evaluation metrics were used to test the model’s
performance, and the results showed strong generalization capability and high accuracy with coefficient of
determination (R2) of 0.997, mean squared error (MSE) of 3.337E-05 kN2, root mean square error of (RMSE)
0.005777 kN, and mean absolute error (MAE) of 0.003633 kN on the test dataset.